Project description
In the course of series production of automotive traction drives, stators in hairpin technology are increasingly coming into focus. Due to the high number of contact points, the laser-based contacting of wire ends plays a major role in hairpin stator production. To realize a stable laser welding process, on the one hand, all influencing variables within the laser welding process itself must be known. On the other hand, relevant interactions with upstream process steps that may have a negative effect on the welding result must be taken into account. Since hairpin stator production is thus characterized by a superposition of numerous non-linear effects, classical methods are reaching their limits when it comes to mapping the causal relationships. Furthermore, there is no fast, cost-effective test method that allows an inline monitoring of the quality of each welded joint, such as the size and number of pores.
In this context, data-driven approaches using the methods of artificial intelligence (AI) are becoming increasingly interesting. Therefore, the project KIKoSA investigates to what extent the quality of welded joints can be monitored using AI based on available material and process data as well as easily integrable sensors. The desired AI solution should enable the detection of deviations in upstream process steps at an early stage and their compensation by an intelligent adaptation of the laser welding process. In this way, this project makes a significant contribution to increasing efficiency in the production of electric drives for electric vehicles.
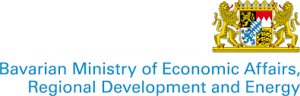
